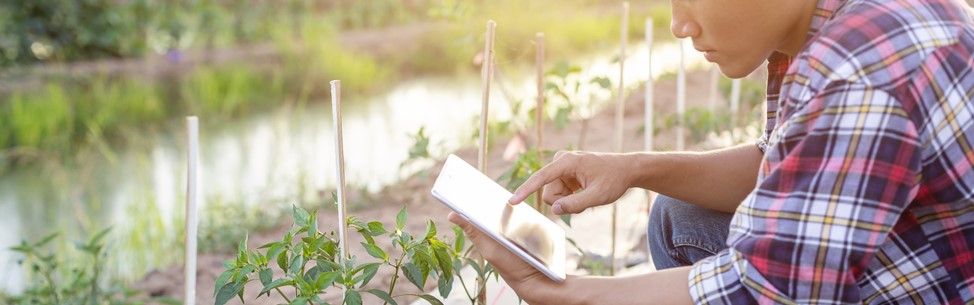
Optimizing Crop Yield Data Collection for Supply Chain Enhancement
Collecting high-quality crop data is expensive, but is paramount to improving policies and programs that can dramatically benefit smallholder farmers in numerous ways, especially by enhancing supply chains. The NASA Harvest Program at the University of Maryland along with their partners collected high-quality datasets to capture yield and other field characteristics in smallholder rice fields in Tanzania. Their local partners included the Sokoine University of Agriculture based in Morogoro and Flamingoo Food Limited. The project leveraged ECAAS’s Open Data Kit (ODK) to collect yield data and test the utility of these data by testing and applying the Global Earth Observations for Crop Inventory Forecasting (GEOCIF) system at a field scale on rice. GEOCIF, a machine learning forecasting model, estimates field-scale crop yields and their applicability to other rice-growing regions. By demonstrating the utility of machine learning models for optimizing yield data collection, this project informed and reduced the cost of collecting yield data that were critical for agricultural decision-making.
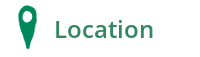
Tanzania

- Project Lead:
- Dr. Catherine Nakalembe
- Team Members:
- Dr. Inbal Becker-Reshef, Dr. Hannah Kerner, Dr. Ritvik Sahajpal
Department of Geographical Sciences, University of Maryland College Park/ NASA Harvest Program
- Dr. Inbal Becker-Reshef, Dr. Hannah Kerner, Dr. Ritvik Sahajpal
- Key Partners:
- Dr. Sixbert K Mourice, Sokoine University of Agriculture (SUA), Tanzania
- Dr. Andreas Schlueter, Adrian Weisensee and Leonard Lusaganya, Flamingoo Foods Company Limited, Tanzania

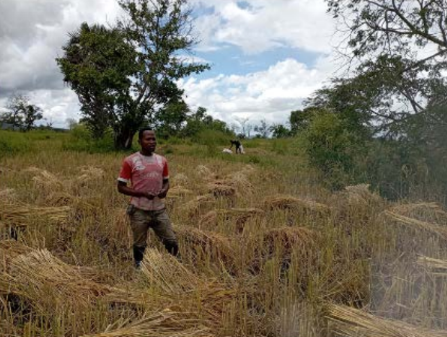
Final Results and Lessons Learned
This project focused on collecting rice yield data through a public-private partnership between NASA Harvest, Flamingoo Foods Limited, and the Sokoine University of Agriculture in Tanzania. Read More about how the project scaled machine-learning-based crop yield forecasting models to estimate rice yield and the generalizability of this model to other rice-growing regions.
February 2023