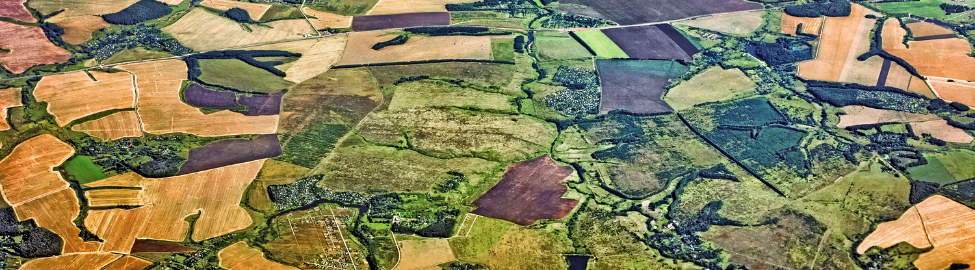
Creating Training Dataset and ML Model to Detect Field Boundaries from Satellite Imagery
Without proper collection, curation, and preparation, data collected on the ground can often lack the details and standards required for use in ML modeling, resulting in a gap in applications, particularly in agricultural monitoring. Radiant Earth Foundation and IDinsight collected data on crop types and field boundaries across five states in India to empower practitioners and stakeholders across developing regions to use machine learning (ML) and earth observation (EO) for agricultural decision-making at both field and national levels.
With the ground reference dataset and a baseline ML model, the Radiant Earth Foundation and IDInsight teams launched the AgriFieldNet competition on the Zindi platform from September to October 2022 to develop benchmark ML Models. A total of 629 individuals enrolled in the competition, with solutions coming from 63 countries. This is the 4th crop recognition challenge Radiant has hosted on Zindi. It had the highest enrollment and participation numbers.
Congratulations to all the participants. The winning solutions are posted for open access on the Radiant MLHub.
1st Place Solution by: Tuo Muhamed, Caleb Emelike, & Taiwo Ogundare
2nd Place Solution by: Mohammad Alasawdah
3rd Place Solution by: MG Ferreira & Tien Dung
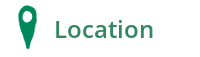
India

Hamed Alemohammad, Radiant Earth Foundation
Amber Myers, Radiant Earth Foundation
Andrew Fraker, IDinsight
Ben Brockman, IDinsight
